It looks like you're new here. If you want to get involved, click one of these buttons!
"Any Means Necessary to Refuse Erasure by Algorithm": Lillian-Yvonne Bertram's Travesty Generator
Abstract: Lillian-Yvonne Bertram's 2019 book of poetry is titled Travesty Generator in reference to Hugh Kenner and Joseph O'Rourke's Pascal program to “fabricate pseudo-text” by producing text such that each n-length string of characters in the output occurs at the same frequency as in the source text. Whereas for Kenner and O'Rourke, labeling their work a “travesty” is a hyperbolic tease or a literary burlesque, for Bertram, the travesty is the political reality of racism in America. For each of the works Travesty Generator, Bertram uses the generators of computer poetry to critique, resist, and replace narratives of oppression and to make explicit and specific what is elsewhere algorithmically insidious and ambivalent. In “Counternarratives”, Bertram presents sentences, fragments, and ellipses that begin ambiguously but gradually resolve point clearly to the moment of Trayvon Martin's killing. The poem that opens the book, “three_last_words”, is at a functional level a near-echo of the program in Nick Montfort's “I AM THAT I AM”, which is itself a version or adaptation of Brion Gysin's permutation poem of the same title. But Bertram’s poem has one important functional difference in that Bertram's version retains and concatenates the entire working result. With this modification, the memory required to produce all permutations of the phrase, “I can’t breathe”, is sufficiently greater than the storage available most computers, so the poem will end in a crashed runtime or a frozen computer--metaphorically reenacting and memorializing Eric Garner’s death. Lillian-Yvonne Bertram's Travesty Generator is a challenging, haunting, and important achievement of computational literature, and in this essay, I expand my reading of this book to dig more broadly and deeply into how specific poems work to better appreciate the collection's contribution to the field of digital poetry.
Question:
Looking back at this essay that first began as a CCSWG post four years ago, I am interested in how Bertram's work (including Travesty Generator and more recent projects) continues to explore computational creativity at the edges of LLM. In a recent If, Then talking about their new chapbook, A Black Story May Contain Sensitive Content, Lillian-Yvonne spoke about the value of "small" and "bespoke" language models and used the phrase "creative research" to frame their project working with a corpus of writing by Gwendolyn Brooks. All this has me thinking about the value of (let's say) artisanal computational poetics as an alternative to LLMs. I have some ideas, but I'll pose this is a question: How and why do tiny language models, "code as text" poetics, and new work built on these legacies critique or resist the operations of large-language models?
Comments
Although the code aspect is largely missing, I think a useful example to bring in here is Jordan Abel's Injun (Talonbooks, 2013). It's a book of 'found' poetry generated from a corpus of 91 public domain (out of copyright) western novels. Abel described his process (which could have been done using automated processes):
"I copied and pasted all 91 western novels into a single Word document and ended up with over 10,000 pages. When I searched that document for the word Injun, I ended up with over 500 results. I copied all of those 500 sentences and pasted them into another Word document where I could read through each sentence individually. The book essentially came together out of me taking a pair of scissors and cutting up each page of that document into a long poem." (CBC website)CBC website
Abel had particular questions he want to ask of this corpus: "'How is this word deployed?' and 'What is it trying to do?' and 'How is it representing and/or misrepresenting Indigenous peoples?' I was interested in looking at all of the contexts in which the word appeared."
So I think Abel's corpus enabled him to ask specific questions and get back concrete, impactful answers about a literary genre's role in developing a racist cultural imaginary around Indigenous peoples. I think the impact of his research and his book would have lost some of its visceral impact if he had not curated a specific corpus. One could imagine his corpus being used to construct a LLM that generated 'western' poetry showing the genre's racism, but I think it wouldn't improve on Abel's 'artisanal' process for doing the same.
I wonder if the utility of SLMs lies not necessarily in resistance against 'large' models but in their explanability and understandability. The scale shift may also allow experimentation with individual units that compose LLMs more broadly, probably (much like suggested here) bringing it closer to code-as-text paradigms for critique. (But Evan's work in the other thread is giving me second thoughts now.)
I would like to respond to Zach's question, first by acknowledging the remarkable contribution his critical code readings of Lillian-Yvonne Bertram's Travesty Generator make to the understanding of "tiny language models" and artisanal computational poetics as an alternative to Large Language Models (LLMs). I was particularly struck by the example of how the operations of “three_last_words” led to computer crash as an "entanglement of technical process and expressive semiotics."
As Jason Boyd notes, we don't have code to look at, largely because A Black Story May Contain Sensitive Content is an exploration of LLMs, which don't make their source code available. However, we can intuit some of their logics and operations based on their output, which is precisely what Bertram set out to do with the works published in this chapbook. As a way to begin to respond to these questions, I want to look at a small sampling from the chapbook, which Bertram offered at the ELO 2022 conference.
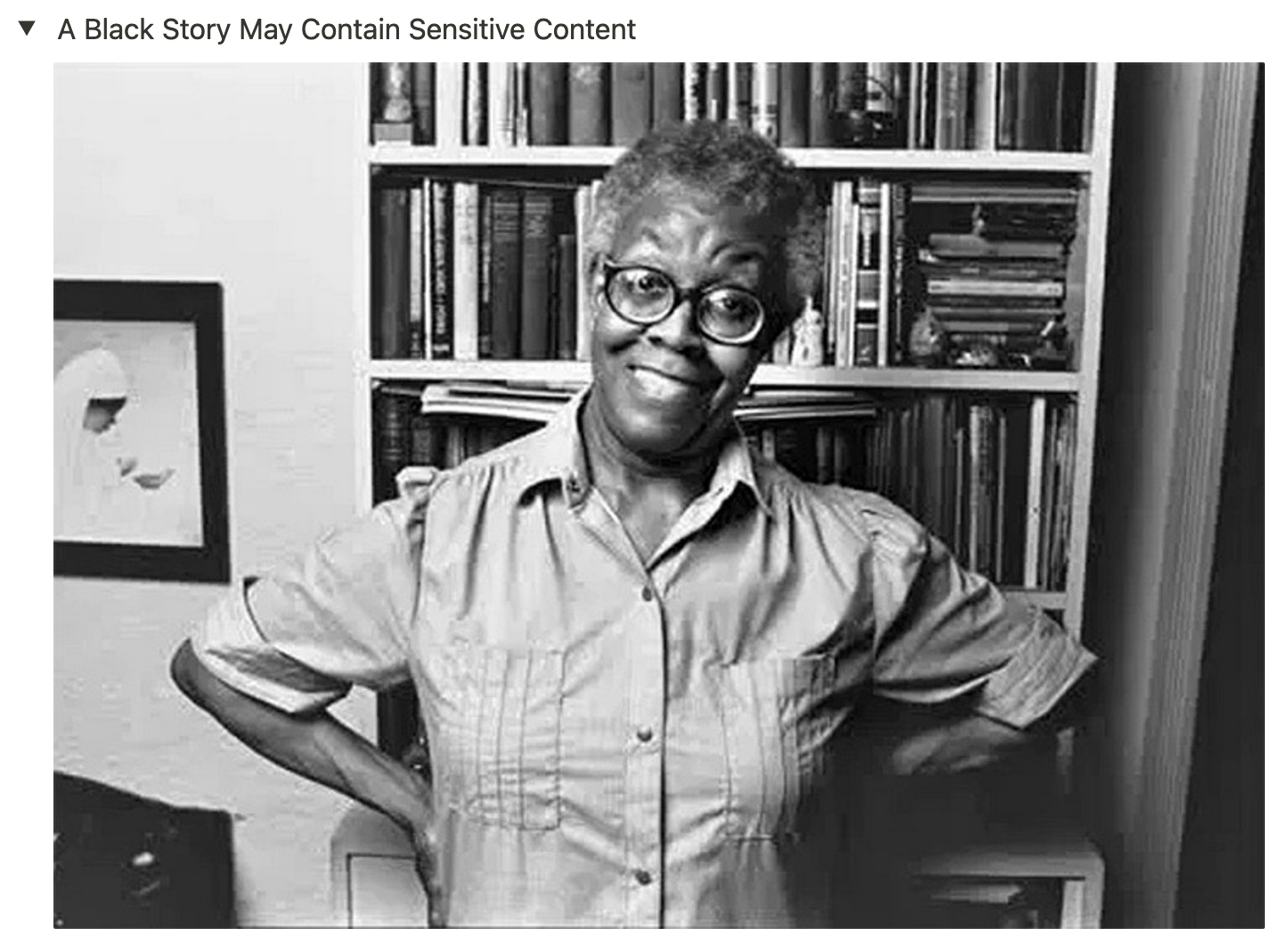
In this sample, available here, we can see how their creative exploration of GPT-3 using Gwendolyn Brooks and the minimalist prompt "tell me a Black story" was like sending a probe into the otherwise impenetrable black box of Open AI's LLM. The experiment consisted first of prompting GPT-3 with the phrase "tell me a Black story" and getting some iterations of its output, then training GPT-3 with texts by Gwendolyn Brooks and repeating the prompt. Here's an example of the out-of-the-box GPT-3 output when presented with this prompt:
Note that GPT-3 readily produced a stereotypical story about racial violence and oppression of a young black girl at the hand of white boys, and how a black man rescues her. Here's another:
This story is also about black pain, oppression, segregation, and offers a supposedly happy ending in which the black family segregates itself into a more accepting community. Both stories offer supposed solutions that reinforce white supremacist narratives of racial strife and segregation, which reveals deeply troubling biases and invites us to ask critical questions about the training data and programming itself for this system.
In contrast, Bertram's Gwendolyn Brooks trained version of GPT-3, which they named Warpland 2.0 (though in the earlier ELO reading version, they referred to it as "G-wendolyn-PT3-Brooks," which I love) produces output that is loving, nurturing, and very much in Brooks' voice. Here's one example:
At a glance, we can read a text that evokes African American literary tradition, love of books, education, reading, and empathy-- a much more positive "black story" than GPT-3 was offering as a default at the time.
Bertram's A Black Story May Contain Sensitive Content features more GPT generated output and can show how the LLM has been trained to avoid what it labels as "sensitive content" (itself a topic worthy of critique). It also shows more of the Warpland 2.0 output, which serves as a powerful counterpoint of what it means to be trained on the work and perspectives on the African American community of such an important poet (Poet Laureate, no less) as Gwendolyn Brooks. Bertram's chapbook also does important work of mapping the closed source, black box operations of LLMs, with prompts and their resulting output serving as a kind of sonar device that we can analyze to intuit its inner logics.
As a way of providing a present day sonar ping of the hidden landscape of GPT-4's programming, I have prompted the Chat GPT version with Bertram's prompt "tell me a Black story." Here's the output:
In reading this fantasy story, I am struck by how safe it is. There is zero engagement with blackness, ethnicity, or any real world issues. It's hard to draw firm conclusions from a single ping, but I suspect the model has been trained to avoid any sensitive content, especially in its Chat GPT instance.
I conclude (for now) with the thought that it is all the more urgent for us to read Bertram's explorations in their chapbook, because of its early mappings of Open AI's LLMs before they were obfuscated by polite countermeasures.
One of the many challenges with LLMs is that they are essentially unknowable. No matter how much you interrogate it via prompt interactions or attempt to trace the weights of different nodes and embeddings, they are so complex and massive that there is no way to create a complete "map" of the internal network or to prod it with any real precision... Given the nature of the training data used and how it's processed, at best we can interrogate these models in a limited way to uncover potential patterns/biases/limitations/proclivities of this specific configuration of the data>statistical weighting>training supervision loop. And the tools we have in order to do this are at the same time controlled, owned, and restricted by the corporations providing access to their models...
Small models or, even better in my view, specific and intentional corpus and algorithm creation (as in the earlier reference to Jordan Abel's work) allow for a level of specificity and interrogability that LLMs will never really have.
Sorry for not adding new impressions, but I completely agree with @emenel in this discussion. By using prompt engineering, it is possible to grasp some of the biases, but still, if we do not know what exactly to ask, we will always miss some of the core parts of the LLM. Reverse engineering is based on finding the right answers to go to the source, but in LLM, there is so much information that this might be a never-ending task. Would it be useful to try to build SLMs based on LLMs?
Coincidentally, Allison Parrish just posted this recent talk that says some of what I was trying to say but better
https://posts.decontextualize.com/language-models-ransom-notes/
https://friend.camp/@aparrish/111998994267165774
Really appreciate the discussion here! I think @ranjodh 's comment resonates most with me, about the value of explainability and understandability in poetics. Instead of an SLM vs. LLM comparison, I find a 'traditional poetry' vs. 'computational poetry' more fruitful.
For me, the question of unknowability doesn't seem like a new one. Poets' brains are classically unknowable black boxes that readers/critics cannot reverse engineer our way into. Very rarely, without direct allusion, can we know the sources of influence in a poem. Algorithmic poetry offers us new insights into process, often embedded into the work itself (thinking of Bertram's #!/usr/bin/env python piece with comment citations).
Prompt engineering seems only able to take us so far, — as Google's Gemini has demonstrated, often counter-biases are built-in to course correct — so for me, I'm more interested in why we want LLMs to give us art at all? Why might we trust them as sources of specific or precise or nuanced stories (or doubt their ability to do so)?
This is something I content with in my diss/what's hopefully being turned into a book eventually. In it, I hope to show how computational poets and artists are using generative computational processes as a strategy for social critique and community building—at this point, all of the case studies I use fall into the category you define, as "artisanal computational poetics."
From a chapter summary: "Through an investigation of combinatorial, computer-generated poems from Lillian-Yvonne Bertram’s 2019 collection Travesty Generator, I show that invention and arrangement are enabled by algorithmic processes, but these processes are deeply social, human ones. For invention, there is a clear authorial hand in the topoi sourced and questions of stasis posed. Bertram’s generative algorithms afford a rhetorical distance from these processes that provide readers with a clearer view of their inner workings. For arrangement, I borrow the “full stack” metaphor from computer engineering to describe how the canon functions in generative, combinatorial texts. Using full stack logic yields a more comprehensive understanding of the rhetorical relationships between authors, sources, readers, and algorithms in Bertram’s generative poems, allowing us to see them as simultaneously individual and collective, attributable to many authors in relation to one another while still working towards the same collective rhetorical end. The algorithmic combination of source corpora in the poems of Travesty Generator showcases the continuities of Black American life, bridging pasts and presents of individual violence and structural oppression with communal confidence in cosmic justice and Black ingenuity."
Also, for those interested in Bertram's most recent chapbook, a recording from the If, Then session on Feb. 16 is up here: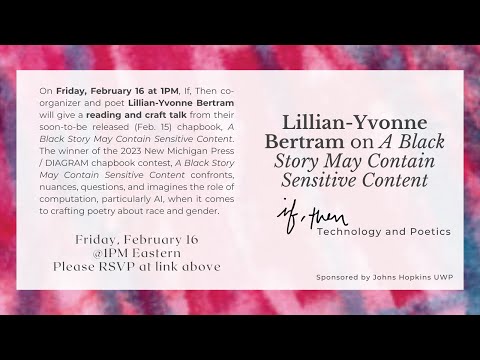